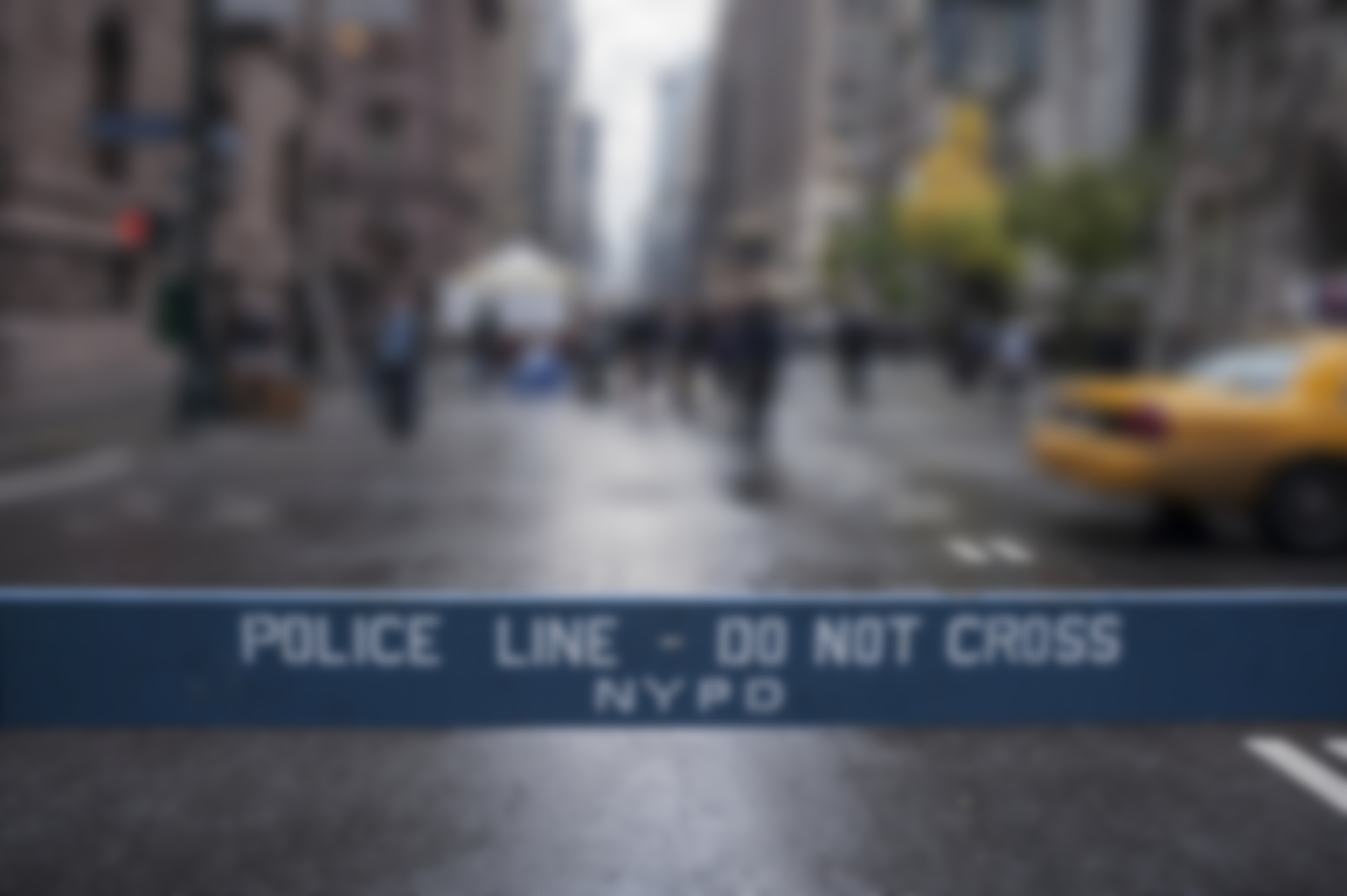
WHAT WE DO
The Public Safety Lab uses the tools of data science and social science to support communities’ efforts to improve both equity and efficiency in public safety outcomes.
Communities and agencies interested in working with the Public Safety Lab can contact us at publicsafetylab@nyu.edu, or follow us at @publicsafetylab.
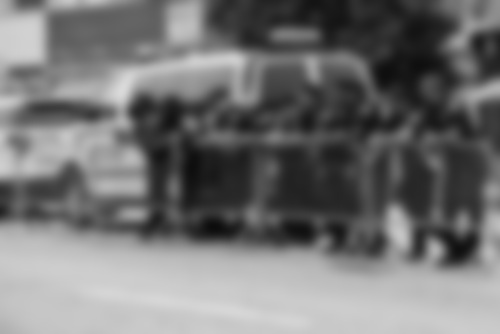
WHO WE ARE
Anna Harvey
Director, Public Safety Lab
Professor of Politics; Affiliated Professor of Data Science and Law
New York University
President, Social Science Research Council
Anna Kyriazis
Post-Doctoral Fellow
Social Science Research Council
Public Safety Lab
New York University
Lauren Schechter
Post-Doctoral Fellow
Social Science Research Council
Public Safety Lab
New York University
Former Contributors
Quincy Alexander
Vidhit Bhargava
Daniel Chain
Anas Jawed
Sylvia Ji
Cora Jung
Aja Klevs
Lyndon Liang
Chutang Luo
Trevor Mitchell
Robert On
Roger Pharr
Adrian Pearl
Antonio Robayo
Evgeniy Shubin
Andrea Wang
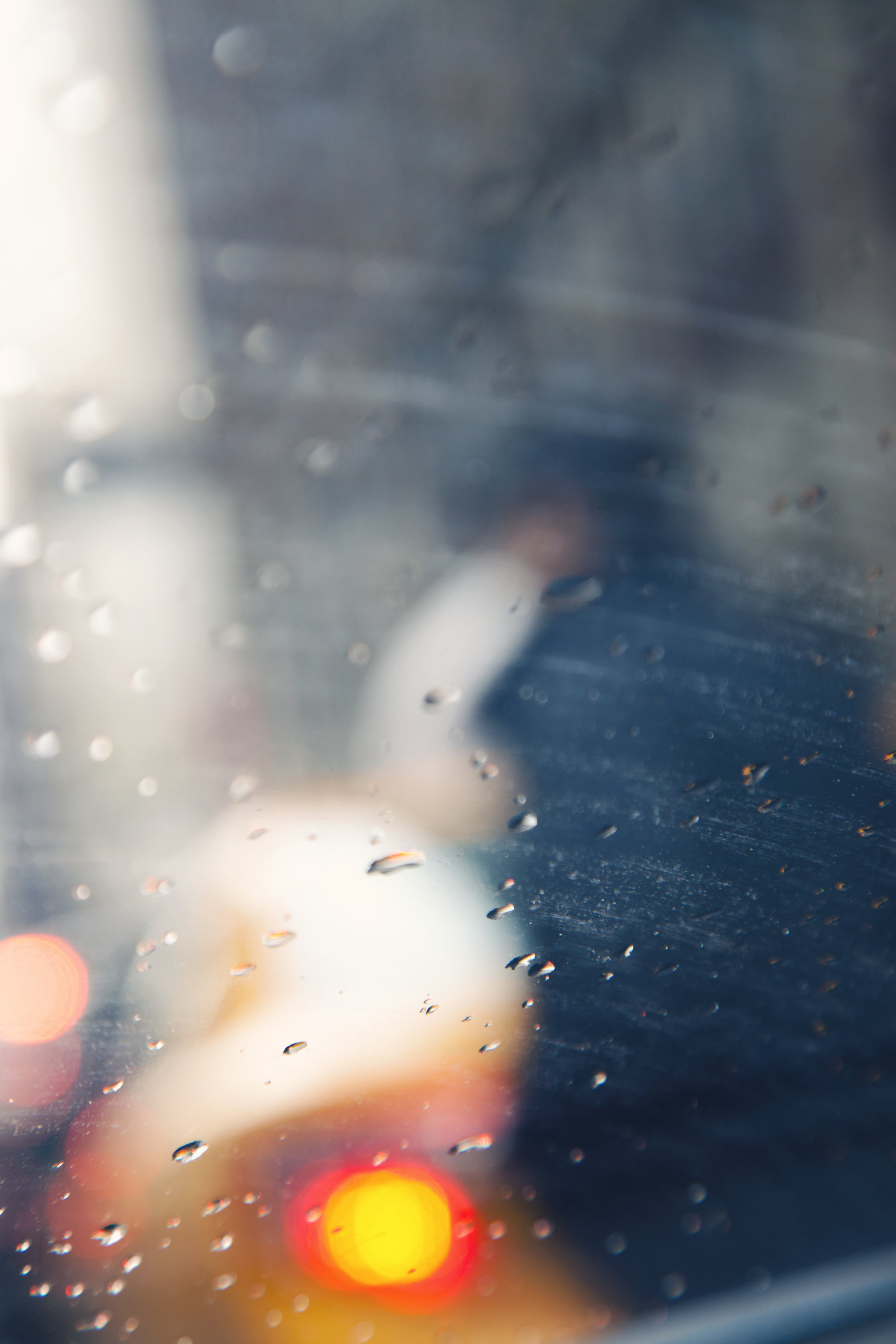
Jail Data initiative
The problem of overincarceration has received considerable attention of late. The focus of this attention, however, has largely been on state and federal prison systems. Often overlooked are local jails. In any given year, county jails will see approximately 11 million new admissions, often because defendants lack the funds to post bail, or even because they lack the funds to pay their fines. Yet we know little about county jail practices, including which counties are systematically jailing defendants pre-trial and/or post-fine, and about the consequences of these practices.
It is possible that longer periods of pre-trial detention, or detainees’ delayed access to counsel, actually increase recidivism, and/or escalate petty offending into more serious offending. Through the generous support of multiple funders, our data science team is scraping daily county jail rosters in over 1,300 counties. Using these data we will be able both to identify counties that are systematically jailing defendants pre-trial and/or post-fine, and to estimate the impacts of these practices on recidivism.
We began collecting daily detainee-level data in September 2019. As we scrape a jail roster, we simultaneously extract multiple structured data fields, which generally include name, date of birth, gender, race/ethnicity, booking charges, bond/bail status, booking date, and release date. We predict charge classes using text fields for booking charges with the help of CJARS at the University of Michigan. We are also able to identify if and when released detainees are rebooked into the same jail.
Our dashboard allows users to visualize daily facility-level data, as well as to request permission to access a restricted database containing daily individual-level data.
The Jail Data Initiative provides daily individual-level data to The Bail Project, and daily facility-level data for approximately 800 jails to the Vera Institute of Justice’s jail population project.
In a recent report commissioned by the Council on Criminal Justice for the National Commission on COVID-19 and Criminal Justice, we analyzed over 14 million daily records on individuals detained between January and July 2020. We found that jail populations began to decrease immediately after the issuance of the White House Coronavirus Guidelines on March 16, reaching a 31% average decrease by May 2. These large decreases in jail populations were achieved both through reductions in admissions, and through releases that exceeded admissions. As jail populations dropped, there were increases in the proportions of detainees who had been booked on felony charges, who were male, who were 25 or younger, and who were Black. These changes in the composition of jail populations persisted even as jail populations began to rise after May 2. We also found that those released from jail just after March 16 were 34% more likely to have been booked on felony charges, and had been detained for 71% longer, relative to those released just prior to March 16. Despite these changes in the composition of those released, there were no differences in 30-, 60-, or 90-day rebooking rates for those released just after March 16, compared to those released just prior. More generally, rebooking rates for jailed individuals released after March 16 remained below pre-pandemic rebooking rates through July. This was true for those booked on felony charges as well as those booked on misdemeanors. Between May 2 and July 20, jail populations rose again, by 12% on average, despite steep increases in new COVID-19 cases in local communities.
In a December 2020 Impact Report Update for the National Commission on COVID-19 and Criminal Justice, we analyzed approximately 19 million individual-level daily jail records collected between January and late October 2020. We found that jail populations have recovered on average 50% of the decreases seen in the early pandemic. Counties that saw higher COVID-19 case growth between March and October 2020 did not see larger jail population decreases. Bookings on misdemeanor and failure to appear charges have decreased, relative to pre-pandemic levels. Although daily bookings remain below pre-pandemic rates, those booked into jail during the pandemic are being detained for approximately twice as long as those booked into jail prior to the pandemic’s onset. To date, 30-, 60-, 90-, and 180-day rebooking rates among those released during the pandemic have remained 13% - 33% below pre-pandemic rebooking rates. To the extent that rebooking rates measure the average public safety risk of releasing individuals from jail, this risk remains lower now than prior to the pandemic. Finally, we found that, while the proportion of Black individuals among daily jail bookings did not change appreciably during the pandemic, the proportion of Black detainees among those released from jails during the pandemic decreased by approximately 5% relative to the pre-pandemic period. As a result, the proportion of jail populations composed of Black individuals rose during the pandemic. All data used in the report is available here.
A New York Times graphics editorial using the Jail Data Initiative data may be found here.
In the first research paper to use the Jail Data Initiative data, we leverage the Jail Data Initiative’s daily individual-level jail records and exploit the timing of incarceration to estimate the causal effects of jail incarceration on voting from jail in 2020. We find that registered voters booked into county jails for the full duration of 2020 voting days were on average 46% less likely to vote in 2020, relative to registered voters booked into the same jails within 7 — 42 days after Election Day. The estimated negative effect of incarceration on voting from jail was much larger for Black registered voters, who were 78% less likely to vote in 2020 if booked into county jails for the full duration of 2020 voting days, relative to Black registered voters booked into the same jails just after Election Day. Placebo tests indicate no effects of 2020 jail incarceration on the 2012 or 2016 turnout of registered voters. We find inconsistent effects of jail incarceration on voter registration in 2020, and effect sizes of comparable magnitude for turnout unconditional on registration status. Our findings reveal the pressing need to enable voting-eligible incarcerated individuals to exercise their constitutional right to vote, and to address troubling racial disparities in the effect of jail incarceration on the exercise of the right to vote.
The Jail Data Initiative is a founding project of Arnold Ventures’ National Partnership for Pretrial Justice, and has also received support from the Chan Zuckerberg Initiative, the Pew Charitable Trusts, the Center for Data Science at New York University, and the Proteus Fund.

prosecutoRial reform initiative
Prosecutors play a vital role in the criminal justice system. After an arrest, prosecutors have numerous choices to make regarding whether and how to pursue prosecution of an offense. In many jurisdictions, prosecutors use this discretion to pursue prosecutions of minor offenses to the full extent of the criminal law. In these jurisdictions, cases involving misdemeanor offenses typically consume a large share of law enforcement, prosecutorial, and judicial resources.
Yet it is unclear whether the aggressive prosecution of misdemeanor offenses actually increases public safety. Aggressive prosecution of misdemeanors may also exacerbate existing inequalities in criminal justice outcomes. Some prosecutors’ offices, however, are pursuing reforms that implement alternatives to prosecution for nonviolent misdemeanor offenses.
With the support of the William T. Grant Foundation, the Chan Zuckerberg Initiative, and Arnold Ventures, we are working with several large district attorney’s offices to understand the causal impacts of misdemeanor prosecution. Misdemeanor cases make up over 80 percent of the cases processed by the U.S. criminal justice system, yet we know little about the causal impacts of misdemeanor prosecution.
A forthcoming article in the Quarterly Journal of Economics reports the first estimates of the causal effects of misdemeanor prosecution on defendants’ subsequent criminal justice involvement. We leverage the as-if random assignment of nonviolent misdemeanor cases to Assistant District Attorneys (ADAs) who decide whether a case should be prosecuted in the Suffolk County District Attorney’s Office in Massachusetts. These ADAs vary in the average leniency of their prosecution decisions. We find that, for the marginal defendant, nonprosecution of a nonviolent misdemeanor offense leads to a 53% reduction in the likelihood of a new criminal complaint and to a 60% reduction in the number of new criminal complaints over the next two years. These local average treatment effects are largest for defendants without prior criminal records, suggesting that averting criminal record acquisition is an important mechanism driving our findings. We also present evidence that a recent policy change in Suffolk County imposing a presumption of nonprosecution for nonviolent misdemeanor offenses had similar beneficial effects, decreasing the likelihood of subsequent criminal justice involvement.
Press: Boston Globe, Chicago Sun-Times, Washington Post, WGBH, WBUR, Commonwealth Magazine, Marginal Revolution, Radio Boston, The Codcast (Commonwealth Magazine podcast), NYU Release, NYU News, Law360, TIME, Reasons to be Cheerful, Probable Causation, New York Times, Atlantic
A second working paper looks more broadly at the election of reform-minded prosecutors who seek to reduce the reach and burden of the criminal justice system. Such prosecutors have implemented reforms such as scaling back the prosecution of nonviolent misdemeanors, diverting defendants to treatment programs instead of punishment, and recommending against cash bail for defendants who might otherwise be detained pretrial. Such policies are controversial, and many worry that they could increase crime by reducing deterrent and incapacitation effects. We use variation in the timing of when these prosecutors took office, across 35 jurisdictions, to measure the effect of their policies on reported crime rates. While our estimates are imprecisely estimated, we find no significant effects of these reforms on local crime rates.
Press: The Economist
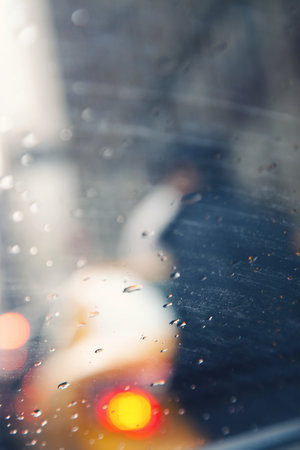
NYPD Officer Misconduct Analysis
In the wake of George Floyd's death at the hands of officers of the Minneapolis Police Department, New York State repealed a 1976 law — known as Section 50-a — that had shielded information about police officer misconduct from public scrutiny. In a report commissioned by Arnold Ventures, we analyzed 323,911 misconduct complaints filed against officers of the City of New York Police Department (NYPD) with the Civilian Complaint Review Board (CCRB), released as a consequence of the repeal of Section 50-a. All data and code used to produce the report's findings are available here.
What we found is disturbing — evidence that the NYPD is more tolerant of excessive misconduct in neighborhoods with more Black residents, and that excessive misconduct in these neighborhoods is associated both with excessive stops, and with officers who accumulate large numbers of misconduct complaints.
We gave the NYPD the benefit of the doubt in our analysis. Our starting premise was that officers’ responses to reported crime have the potential to escalate into misconduct complaints. We therefore expected precincts with higher reported crime volume to have higher misconduct complaint volume. That turned out to be true. After mapping approximately 7 million crimes reported between 2006-2019 in New York's 77 precincts and 11 transit districts to the misconduct complaints made against officers assigned to those commands, we found that higher reported crime volume was associated with higher misconduct complaint volume, both for all complaints and for those complaints found to be "substantiated" by the CCRB.
However, even after adjusting for reported crime volume, many precincts still had “excess” misconduct — higher numbers of complaints and substantiated complaints than one would predict, given reported crime volume. As we looked at this "excess" misconduct, troubling patterns emerged.
First, precincts with larger proportions of Black residents had higher levels of excess misconduct complaints, while precincts with larger proportions of non-Hispanic white and Asian residents had lower levels of excess misconduct complaints.
For example, Precinct 75 in Brooklyn (East New York) had the highest rate of excess or unexplained misconduct complaints, averaging 38 more misconduct complaints per year than predicted by its reported crime volume, for an observed rate of misconduct complaints 31% higher than the predicted rate. In the 2010 Census, Precinct 75 was recorded as having 60% Black residents, 3% non-Hispanic white residents, and 5% Asian residents. By contrast, Precinct 122 on Staten Island had one of the lowest rates of excess misconduct complaints, averaging 22 fewer complaints per year than predicted by its reported crime volume, for an observed rate of misconduct complaints 36% lower than the predicted rate. In the 2010 Census, Precinct 122 was recorded as having 3% Black residents, 79% non-Hispanic white residents, and 7% Asian residents. These patterns held throughout the data, both for all complaints and for those complaints found to be substantiated by the CCRB.
Second, after mapping the approximately 4 million stops made by NYPD officers between 2006-2019 to the misconduct complaints, we found that precincts with more excess misconduct also had more excess stops, or higher numbers of stops than one would predict, given reported crime volume. Residents of Precinct 75, the precinct with the highest level of excess misconduct, experienced approximately 4,000 more pedestrian stops per year than predicted between 2006 and 2019. Residents of Precinct 122 experienced approximately 3,500 fewer pedestrian stops than predicted over the same period. This pattern held throughout the data, both for all complaints and for those complaints found to be substantiated by the CCRB.
Third, precincts with more excess misconduct tolerated officers who accumulated more misconduct complaints. In Precinct 75, the precinct with the highest level of excess misconduct, an officer accused of misconduct between 2006-2019 had on average 6.4 complaints brought against him during that period. Officers accused of misconduct in Precinct 122 averaged 38% fewer complaints per accused officer over the same period. This pattern held throughout the data, both for all complaints and for those complaints found to be substantiated by the CCRB.
Fourth, these findings were related. Precincts with larger proportions of Black residents (like Precinct 75) tolerated not only disproportionately high levels of excess officer misconduct, but also disproportionately high levels of excess stops and misconduct committed by disproportionately fewer officers. Again, these patterns held throughout the data, both for all complaints and for those complaints found to be substantiated by the CCRB.
New Yorkers have a right to expect policing that is both responsive and fair. But the patterns revealed by our analysis indicate that how a neighborhood is policed depends on how many of its residents are Black. There are more incidents of officer misconduct unrelated to a precinct's reported crime volume, more stops unrelated to a precinct's reported crime volume, and misconduct that is committed by fewer officers, in precincts with more Black residents.
Click on the image to the left to access the Public Safety Lab NYPD Officer Misconduct Analysis. Data and code available here.
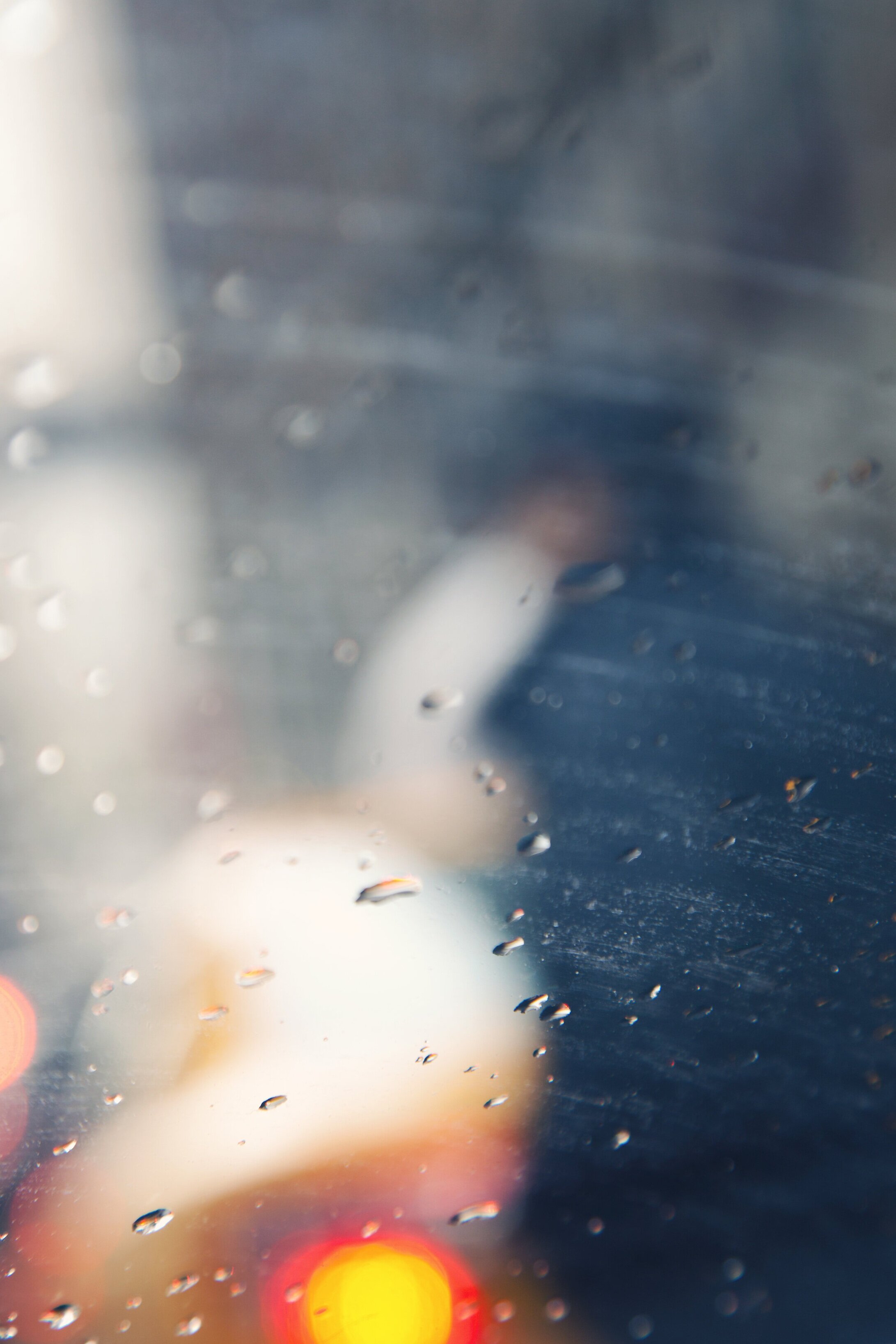
CURRENT Projects
Policing for Revenue
In recent years numerous observers have raised concerns about “policing for profit,” or the deployment of law enforcement resources to raise funds for cash-strapped jurisdictions. However, identifying the causal effect of fiscal incentives on law enforcement behavior has remained elusive. This project leverages a discontinuity in the rules allocating fine revenue from traffic citations issued by a large highway patrol agency, finding that the frequency and severity of traffic accidents increase sharply just above the threshold reducing the share of fine revenue captured by the agency. Cited drivers in towns just below this threshold are also given fewer days to pay their fines and are less likely to pay their fines on time, leading to higher risks of late fees and license suspension. These findings suggest that fiscal concerns can in fact impact public safety decisions, and can lead to distortions in public safety outcomes. (PAPER HERE)
Discrimination in Criminal Appeals
Using content extracted from the scraped text corpus of the approximately 38,000 slip opinions in criminal appeals heard by New York State's intermediate appellate courts between 2003-2017, appellate judge election and appointment data sourced from the New York State Board of Elections and the New York State Judicial Screening Committee, and defendant demographic and conviction data scraped from the New York State Department of Corrections' inmate database, we report the first within-judge estimates of the effects of both reelection and reappointment incentives on judicial votes on criminal appeals. Our findings indicate that impending judicial reappointment induces a 49 - 52\% within-justice decrease in pro-defendant votes in appeals involving Black defendants heard by all-white panels, but has no effects on votes in other cases. We find no additional effects of impending reelection on appellate justice votes in criminal appeals. Our findings suggest the need for greater attention devoted both to potential selection effects, and to heterogeneous effects by defendant and judge race, in studies of judicial retention institutions. (PAPER HERE)
Discrimination in Policing
Black Americans are substantially less safe than white Americans, with persistently higher risks of crime victimization. One possible cause of racial disparities in crime victimization may lie in racially disparate law enforcement responses to crime experienced by Black and white victims. We leverage idiosyncratic variation in the litigation of law enforcement agencies for racially discriminatory employment practices to identify changes in the nature of the police response to Black crime victimization. Using data from the National Crime Victimization Survey between 1979 and 2004, and a series of estimators appropriate for difference-in-differences designs with staggered treatment, we find that litigation over racially discriminatory employment practices in law enforcement agencies decreased Black crime victimization by magnitudes ranging between 24 - 27%, but had no discernible impacts on white crime victimization, reducing the pretreatment racial gap in crime victimization by 73 - 82%. Decreases in Black crime victimization appear in the first year after litigation onset, consistent with efforts by litigated departments to address racial disparities in the police response to reported crime. (PAPER HERE; PROBABLE CAUSATION PODCAST EPISODE HERE)
Misdemeanor Prosecution
Communities across the United States are reconsidering the public safety benefits of prosecuting nonviolent misdemeanor offenses, yet there is little empirical evidence to inform policy in this area. In this paper we report the first estimates of the causal effects of misdemeanor prosecution on defendants’ subsequent criminal justice involvement. We leverage the as-if random assignment of nonviolent misdemeanor cases to Assistant District Attorneys (ADAs) who decide whether a case should be prosecuted in the Suffolk County District Attorney’s Office in Massachusetts. These ADAs vary in the average leniency of their prosecution decisions. We find that, for the marginal defendant, nonprosecution of a nonviolent misdemeanor offense leads to a 53% reduction in the likelihood of a new criminal complaint and to a 60% reduction in the number of new criminal complaints over the next two years. These local average treatment effects are largest for defendants without prior criminal records, suggesting that averting criminal record acquisition is an important mechanism driving our findings. We also present evidence that a recent policy change in Suffolk County imposing a presumption of nonprosecution for nonviolent misdemeanor offenses had similar beneficial effects, decreasing the likelihood of subsequent criminal justice involvement.
Quarterly Journal of Economics (forthcoming)
Press: Boston Globe, Chicago Sun-Times, Washington Post, WGBH, WBUR, Commonwealth Magazine, Marginal Revolution, Radio Boston, The Codcast (Commonwealth Magazine podcast), NYU Release, NYU News, Law360, TIME, Reasons to be Cheerful, Probable Causation, New York Times, Atlantic
Prosecutorial Reform and Local Crime Rates
Many communities across the United States have elected reform-minded, progressive prosecutors who seek to reduce the reach and burden of the criminal justice system. Such prosecutors have implemented reforms such as scaling back the prosecution of nonviolent misdemeanors, diverting defendants to treatment programs instead of punishment, and recommending against cash bail for defendants who might otherwise be detained pretrial. Such policies are controversial, and many worry that they could increase crime by reducing deterrent and incapacitation effects. In this paper we use variation in the timing of when these prosecutors took office, across 35 jurisdictions, to measure the effect of their policies on reported crime rates. While our estimates are imprecisely estimated, we find no significant effects of these reforms on local crime rates.
Press: The Economist
Voting From Jail
We leverage new data on daily individual-level jail records and exploit the timing of incarceration to estimate the causal effects of jail incarceration on voting from jail in 2020. We find that registered voters booked into county jails during 2020 voting days for the full duration of voting days were on average 46% less likely to vote in 2020, relative to registered voters booked into the same jails within 7 -- 42 days after Election Day. The estimated negative effect of incarceration on voting from jail was on average 46% larger for Black registered voters, relative to white registered voters. Black registered voters booked into county jails during 2020 voting days for the full duration of voting days were on average 78% less likely to vote in 2020, relative to Black registered voters booked into the same jails just after Election Day. Placebo tests indicate no effects of 2020 jail incarceration on 2012 or 2016 turnout. Our findings reveal a pressing need to enable voting-eligible incarcerated individuals to exercise their constitutional right to vote.
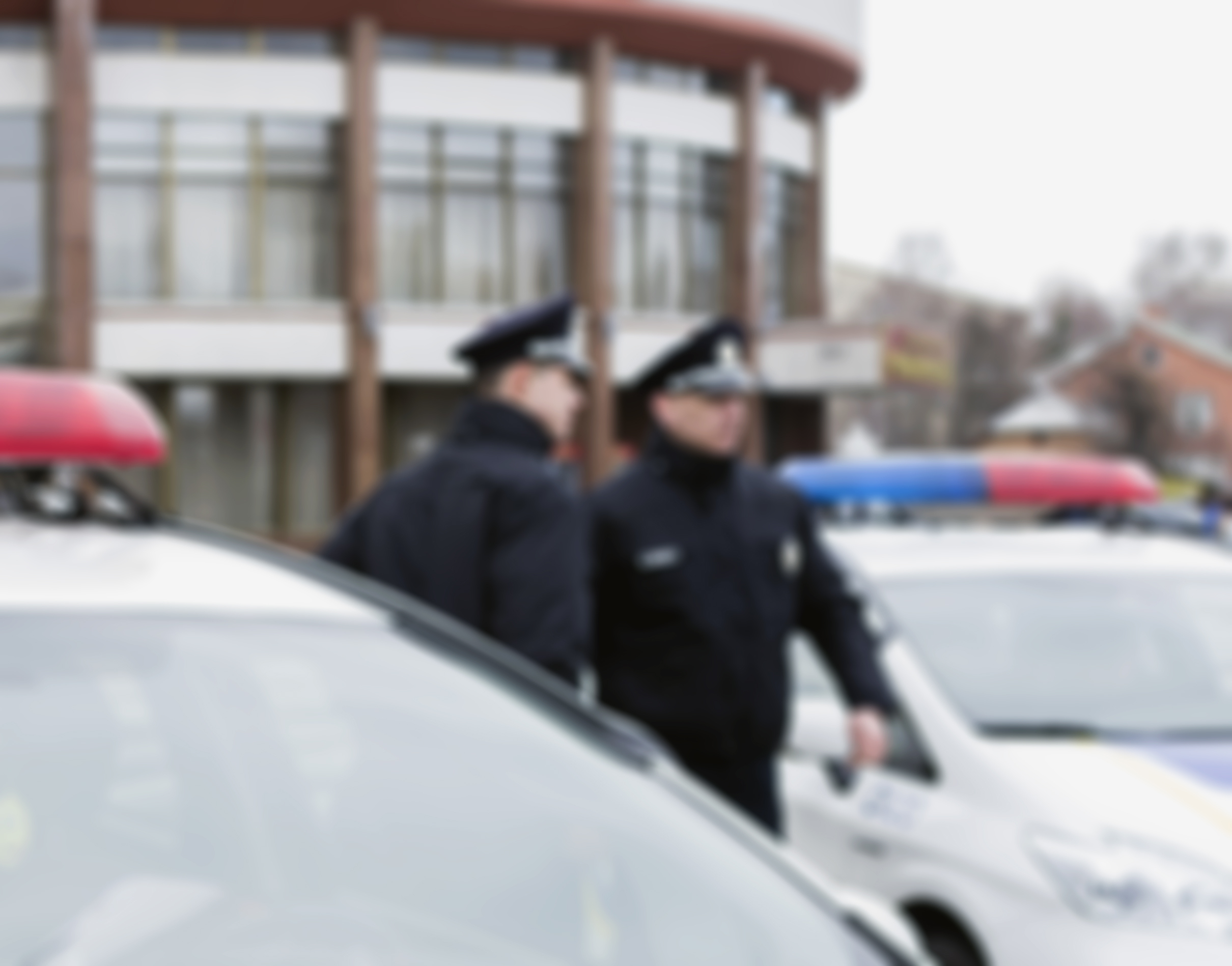
2019 Workshop on Data-Driven Criminal Justice Reform
Data-Driven Prosecution
Office of Suffolk County District Attorney Rachael Rollins
The Justice Collaboratory, Yale Law School
Public Safety Lab, New York University
Tuesday August 20th 2019 9 AM-5 PM
Jurow Hall, New York University
Silver Center for Arts and Science
8:30-9:00: Light breakfast available
9:00-9:30: Opening remarks
Bobby Constantino, Chief of Innovation & Strategy, Office of Suffolk County District Attorney Rachael Rollins
Caroline Sarnoff, Executive Director, Justice Collaboratory at Yale Law School
Anna Harvey, Professor of Politics, Affiliated Professor of Law, and Director, Public Safety Lab, New York University
9:30 - 10:30
Matthew Saniie, Chief Data Officer, Cook County State’s Attorney’s Office
Oren Gur, Director of Research and Policy Advisor, Philadelphia District Attorney’s Office
Jessica Valarezo, Policy and Planning Analyst, Manhattan District Attorney’s Office
10:30-11:30
Alyson Grine, Assistant District Attorney, Durham District Attorney’s Office, District 16
Branden Dupont, Business Analyst, Institute for Health & Equity, Medical College of Wisconsin
Meg Reiss, Chief of Social Justice, Brooklyn District Attorney's Office
11:30-12:30
David Kilmer, Director of Data Engineering, Measures for Justice
Dharmesh Tarapore, Technical Director, BU Spark!, Hariri Institute for Computing, Boston University
Kaiton Williams, Product Developer, Promise
Nasser Eledroos, Technologist, Office of Suffolk County District Attorney Rachael Rollins
12:30-1:30: Lunch
1:30-2:45
Josi Nielsen, Communications Director, Wesley Bell, Prosecuting Attorney, St. Louis County
Tom Tyler, Professor of Law and Founding Director of The Justice Collaboratory, Yale Law School
Robert On, Chan Zuckerberg Initiative
2:45-4:00
Cynthia Conti-Cook, Staff Attorney, The Legal Aid Society
Ivan Calaff, Lab Member, Center for Justice, Columbia University
Katy Naples-Mitchell, Legal Fellow, Charles Hamilton Institute for Race & Justice, Harvard Law School
Tiffany Caban, Candidate for Queens County's District Attorney
4:00-5:00: Wrap-up lightning round
Participating Organizations
Arnold Ventures
Boston University BU Spark!
Brooklyn District Attorney's Office
Chan Zuckerberg Initiative
Columbia University Center for Justice
Court Watch
Cook County State’s Attorney’s Office
District of Columbia Attorney General's Office
Durham District Attorney's Office
Fair and Just Prosecution
Harvard Law School Charles Hamilton Houston Institute for Race & Justice
Manhattan District Attorney’s Office
Measures for Justice
Medical College of Wisconsin Institute for Health and Equity
Milwaukee County District Attorney’s Office
MIT Media Lab Human Dynamics Group
New York University Public Safety Lab
Northeastern University Center on Crime and Community Resilience
Parisa Tafti Campaign, Arlington County Commonwealth's Attorney
Philadelphia District Attorney's Office
Promise
San Francisco District Attorney's Office
St. Joseph's College
St. Louis County Prosecuting Attorney's Office
Suffolk County District Attorney's Office
The Legal Aid Society
Tiffany Caban Campaign, Queen's County District Attorney
University of Chicago Crime Lab
University of Missouri St. Louis Community Innovation and Action Center
Upturn
William T. Grant Foundation
Yale Law School Justice Collaboratory
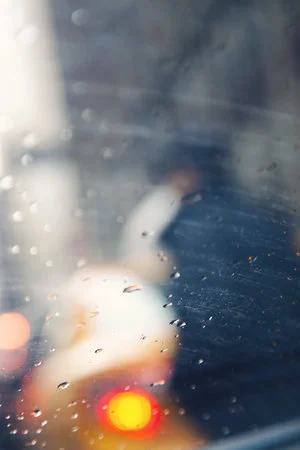
2018 Workshop on Data-Driven Criminal Justice Reform
Public Safety Lab, New York University
Friday October 19th 2018 9 AM-4:30 PM
Saturday October 20th 2018 9 AM-1 PM
Lester Pollack Colloquium Room
Furman Hall, New York University School of Law
Data science and social science may offer opportunities to support communities’ efforts to pursue criminal justice reform. For example, many relatively minor offenses are easy to detect and interdict. Many more serious offenses are much harder to detect and interdict. As a consequence we may overpunish relatively minor offenses, and underpunish more serious offenses. Data science and social science strategies may allow us to achieve more socially productive allocations of criminal justice resources.
This workshop will convene university researchers along with representatives from public agencies and nonprofit organizations to engage in a broad-ranging conversation on data-driven criminal justice reform.
Space is limited and registration is required; please rsvp here if you wish to attend. Rsvps will close when capacity is reached.
Friday, October 19
8:30-9:00: Light breakfast available
9:00-9:15: Opening remarks
9:15-10:45: Do we overpolice minor offenses?
A significant share of law enforcement effort is directed at policing relatively minor offenses, such as infractions, violations, and misdemeanors. Law enforcement personnel are often asked to respond to situations that might better be handled by medical or social service professionals. How are these resource allocation decisions made? Are they effective? Are they equitable? What consequences do they have? Are there better ways to make these decisions? What kinds of data would we need to answer these questions?
Wesley Bell, Democratic Candidate for St. Louis County Prosecutor
Jenn Rolnick Borchetta, Deputy Director, Impact Litigation, The Bronx Defenders
Captain Clay Farmer, North County Police Cooperative, St. Louis County
Ravi Shroff, Assistant Professor of Applied Statistics and Urban Informatics, New York University
11:00-12:30: Do we underpolice more serious offenses?
Many more serious offenses, such as gun violence, gun trafficking, drug trafficking, and sex trafficking, are difficult to detect and/or seemingly intractable. Recent developments in data science may offer tools to help law enforcement agencies address these more serious offenses. How do these predictive policing tools work? Are they effective? Are they equitable? What consequences do they have? What kinds of data would we need to answer these questions?
Anna Harvey, Professor of Politics and Director, Public Safety Lab, New York University
Justin McCrary, Paul J. Evanson Professor of Law, Columbia Law School
Daniel Neill, Associate Professor of Computer Science and Public Service, New York University
Chief Lori Pollock, Crime Control Strategies, New York Police Department
12:30-1:30: Lunch
1:30-3:00: Is pretrial decision making optimal?
A number of important decisions are made during the period between arrest and the disposition of a defendant’s case. For example, prosecutors make decisions over whether to pursue prosecution, the nature and count of charges brought, bail and detention recommendations, and plea offers. Judges make bail decisions, rule on pretrial motions, and approve or disapprove of plea bargains. How are these decisions made? What consequences do they have? What kinds of data-driven strategies might assist prosecutorial decision making? Do bail algorithms offer the promise of more effective bail decisions? What kinds of data would we need to answer these questions?
Sharad Goel, Assistant Professor of Management Science and Engineering, Executive Director, Stanford Computational Policy Lab, Stanford University
Scott Levy, Special Counsel to the Criminal Defense Practice, The Bronx Defenders
Jens Ludwig, McCormick Foundation Professor of Social Service Administration, Law, and Public Policy; Director, University of Chicago Crime Lab
Maria McKee, Principal Analyst, Office of San Francisco District Attorney
3:15-4:30: Access to counsel
Jurisdictions vary widely in their practices with respect to providing counsel to the indigent. What factors affect access to counsel? How does access to counsel affect outcomes? How does the quality of counsel affect outcomes? Are there data-driven strategies to promote more effective access to counsel? What kinds of data would we need in order to answer these questions?
Amanda Agan, Assistant Professor of Economics and Affiliated Professor of Criminal Justice, Rutgers University
Andrew Davies, Director of Research, New York State Office of Indigent Legal Services
Miguel de Figueiredo, Associate Professor of Law and Terry J. Tondro Research Scholar, University of Connecticut
Saturday, October 20
8:30-9:00: Light breakfast available
9:00-10:15 Incarceration and reentry
Incarceration is expensive, both in terms of its direct financial costs and in terms of the indirect costs it imposes on defendants post-release. Is incarceration an effective strategy to achieve deterrence? Is it equitable? What consequences does it have? Are there more cost-effective alternatives that can achieve both greater deterrence and greater reentry success? What kinds of data would we need to answer these questions?
Monica Deza, Assistant Professor of Economics, Hunter College
Elizabeth Glazer, Director, Mayor’s Office of Criminal Justice, New York City
Jennifer Skeem, Mack Distinguished Professor of Social Welfare and Public Policy, University of California, Berkeley
10:30-11:45 Transparency and accountability
Recent media attention directed at police shootings, instances of officer misconduct, and civilian complaints has raised questions about the availability and accuracy of law enforcement data. What kinds of data should law enforcement agencies report to the communities they police? What consequences, both positive and negative, might follow from reporting these data? How would we know?
Cynthia Conti-Cook, Staff Attorney, Criminal Special Litigation Unit, Legal Aid Society, New York City
Jonathan Mummolo, Assistant Professor of Politics and Public Affairs, Princeton University
Anita Ravishankar, Fellow, The Lab @ DC
12:00-1:00: Working Lunch: Developing strategies for data-driven criminal justice reform
In this final session we will review the most promising strategies identified to promote data-driven criminal justice reform, discuss potential partnerships, and develop a roadmap for future research.
Chris Dawes, Associate Professor of Politics, New York University
Sharad Goel, Assistant Professor of Management Science and Engineering, Executive Director, Stanford Computational Policy Lab, Stanford University
Jonathan Mummolo, Assistant Professor of Politics and Public Affairs, Princeton University
Daniel Neill, Associate Professor of Computer Science and Public Service, New York University

Gallery
Data Science and Criminal Justice Reform; Anna Harvey, Director, Public Safety Lab;
University of Louisville, January 2018
Workshop on the Economics of Risky Behavior, Cortes, Corsica, June 2018;
American University School of Public Affairs; co-sponsored by Public Safety Lab
The New Technology of Policing; George Mason University October 2018
Jonathan Klick, Professor of Law, University of Pennsylvania; Anna Harvey, Professor of Politics and Director, Public Safety Lab, New York University; Desmond Patton, Associate Professor of Social Work, Columbia University; Sean Goodison, Deputy Director, Police Executive Research Forum; Hon. Judge Albert Diaz, United States Court of Appeals for the 4th Circuit
2019 Public Safety Lab Workshop on Data-Driven Prosecution, August 2019, NYU
Featuring representatives from 11 district attorney’s offices, nonprofit organizations, foundations, and the research community; co-sponsored by the Office of Suffolk County District Attorney Rachael Rollins and The Justice Collaboratory at Yale Law School
Minds and Machines: A Dialogue About the Relationship Between Humans and Artificial Intelligence; New York University, December 2019
Incarceration and COVID-19: NYU Wagner Seminar Series, April 28, 2020
